UltraLiDAR: Learning Compact Representations for LiDAR Completion and Generation
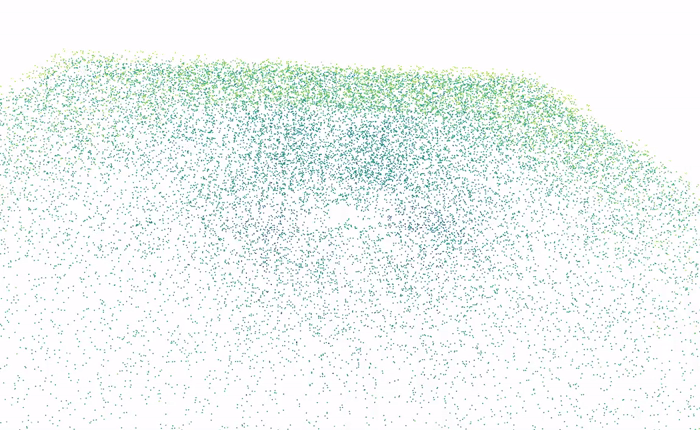
Abstract
Overview
UltraLiDAR learns discrete representations from large-scale LiDAR point clouds and performs realistic, scalable and controllable LiDAR completion and generation. Top row: Sparse-to-dense LiDAR completion; Second row: Controllable manipulation of real LiDAR with actor removal and insertion; Third row: Diverse LiDAR generation with realistic global structure and fine-grained details; Bottom row: Conditional scene generation with partially observed point clouds.
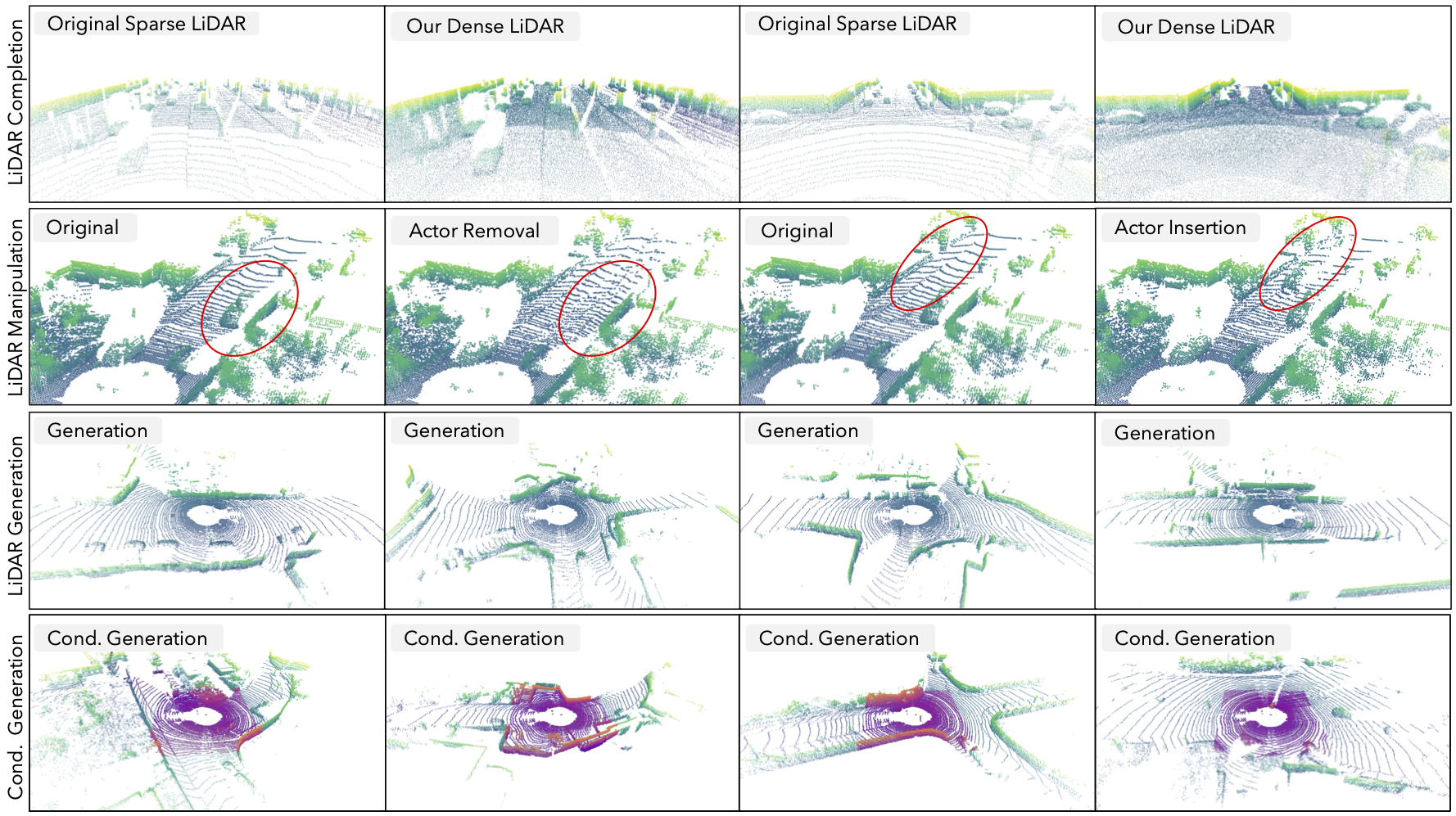
Video
Play with sound.
Sparse-to-Dense LiDAR Completion
Our densified point clouds preserves the original structure of the sparse input and is able to recover the geometry of the vehicles. The results are consistent across time even if we reconstruct each frame individually.
Unconditional LiDAR Generation
Method
For (a) LiDAR completion, the sparse encoder maps the sparse point cloud to discrete codes, and the dense decoder reconstructs dense data from them; For (b) LiDAR generation, the transformer model starts from a blank canvas or canvas with codes mapped from the partial observations; and iteratively predicts and updates the missing parts. The decoder produces the LiDAR output given the predicted code as the generation results.

Comparison with other methods
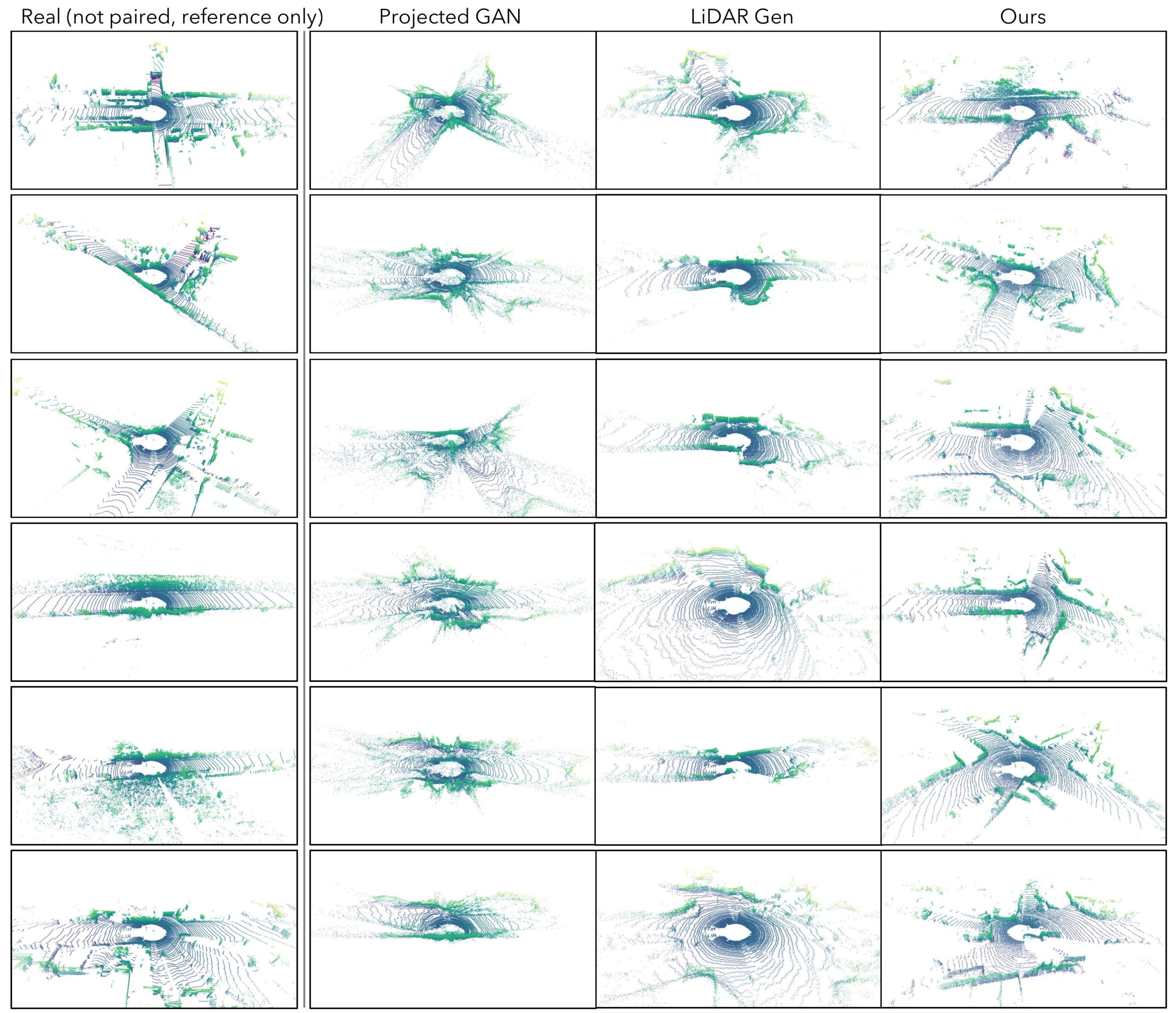
We plan to release the results we used for A/B test to facilitate future research.
Generation Results
We show some conditional and unconditional generation results from the model trained on KITTI-360 data.
BibTeX
@inproceedings{xiong2023learning,
title = {Learning Compact Representations for LiDAR Completion and Generation},
author = {Xiong, Yuwen and Ma, Wei-Chiu and Wang, Jingkang and Urtasun, Raquel},
booktitle = {CVPR},
year = {2023},
}